How to synthesise real-world evidence and AI to transform patient-driven healthcare
Pharmaceutical companies have more real-world data (RWD) than ever
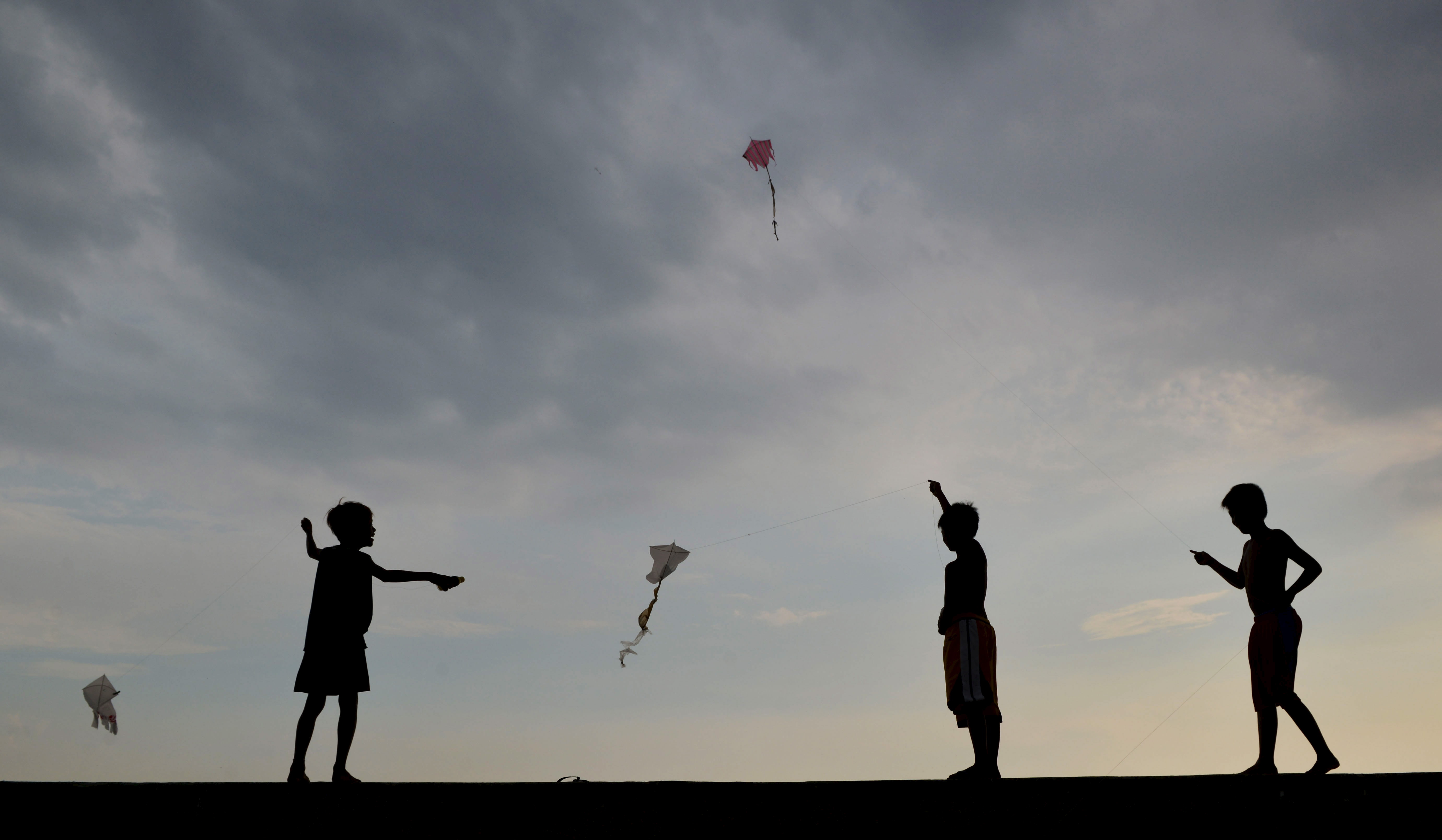
As health records have gone digital, sensors have enabled round-the-clock patient monitoring, and other changes have increased the availability of RWD, the challenge has evolved from accessing information to turning data into insights. AI offers pharma a way to tame the complexity—if companies can manage challenges related to streaming data into standardised models, data quality, and interpretation.
Analysing RWD to generate real-world evidence (RWE) delivers value across the value chain, from drug discovery through to market access and adherence. Pharma companies need to standardise, organise, and interpret the data at scale to realise those benefits. With the scale overwhelming manual processes, AI is emerging as a key enabler of the use of RWE.
How AI is enhancing RWE
Talking at an online Reuters event, Andrew Rosen, Senior Director, Integrated Evidence Team Lead at Moderna, identified “at least three major steps forward” that could be enabled by the combination of AI and RWE.
“One is personalised medicine through data, real-world-evidence, and AI. We can enable better clinical decision making, treating patients not just as their disease, but the complete constellation of properties. Clinical, demographic, personal and behavioural, even socio economic [elements], can all be factored in to recommend an approach to treatment that's very specific to that patient,” Rosen said.
Rosen named data and models that enable early disease detection and empower physicians to clearly show patients why they are advocating a treatment as other improvements that AI and RWE can enable.
Nicholas Kelley, Director for Data Science and AI Portfolio Strategy and Innovation at Novartis, provided a real-world example to illustrate another use case. Using social listening, the team identified a potential problem with the dosing of a biologic. The issue was common knowledge and a topic of discussion in the patient community, but little known among physicians.
The example shows how RWE can capture the patient voice. Kelly Zou, Head Of Global Medical Analytics and Real World Evidence at Viatris, expanded on the idea of AI as a tool for patient-centricity, discussing how the application of the technology to RWE benefits patients by accelerating drug development and improving access.
Why data quality is critical
The AI applications are supported by diverse sets of RWD. Working with everything from claims data to wearable sensor readings, pharma companies must understand how to assess the quality of datasets and ensure they are suitable for AI-enabled interpretation.
“AI models are going to live and die by the quality of the data,” Rosen said. That means accessing data that is current, comprehensive, and coded in a consistent way. It also means ensuring technical solutions to create data lakes, support data governance, and enable analysis are in place. As Rosen said, there are “a wealth of different types of models and methods to choose from,” so applying “the right one, as per the questions or solutions that you're working toward, [is] absolutely critical.”
Gilles Paubert, Senior Vice President, Global Head at Cegedim Health Data, emphasised the importance of clearly specifying the medical problem and context before starting AI development. “Clinical success criteria must be well defined and assessment of the potential risk associated with prediction error must be carried out,” Paubert said, adding that it is very important to work with the most recent data possible. “A reality from a year ago is sometimes no longer a reality,” the Cegedim SVP said.
The age of the data is easy to assess but evaluating other criteria is more challenging. As Zou explained, quality assessment is very complex because data comes in “all forms and shapes.” Most data collected in the real world is unstructured and some forms of information, such as images, cannot be evaluated using traditional quality metrics. Zou outlined how regulators are thinking about the challenge.
“The European Medicines Agency has a draft guidance. They actually put down reliability, extensiveness, coherence, timeliness, and relevance as the five dimensions. There are multiple sub-dimensions,” Zou said. “The FDA talks about completeness, consistency, and accuracy. There are other frameworks such as Sentinel. How would you harmonise these kinds of concepts across different regulatory agencies?”
Zou added that pharma companies can look to the International Council for Harmonisation of Technical Requirements for Pharmaceuticals for Human Use for guidance on how to approach data quality and AI.
Showing the value of AI models
Regulators are one of several groups of stakeholders that need convincing of the quality of RWE and AI. To win over physicians, Rosen said companies must both “demonstrate the reliability of the data and the models” and show the approaches “can actually enhance clinical care.”
Rosen gave an example of how the personalisation of data has driven change, explaining that providing “hands-on access to the real-world evidence” and allowing physicians to “filter and work with the data” increases confidence. Rather than sharing “generic data or stale disease state decks,” medical affairs can present personalised or hyperlocal RWE. Rosen has seen the approach “galvanise action.”
The example points to how analysis of RWE can reveal deeper layers of meaning to provide benefits across the value chain. As the volume of data increasingly overwhelms capacity to sort and interpret it, the synthesis of RWE and AI will be central to efforts to cope with the complexity and unleash the value of real-world data.