When the Machines Take Over
How technology is making the world's health data useful
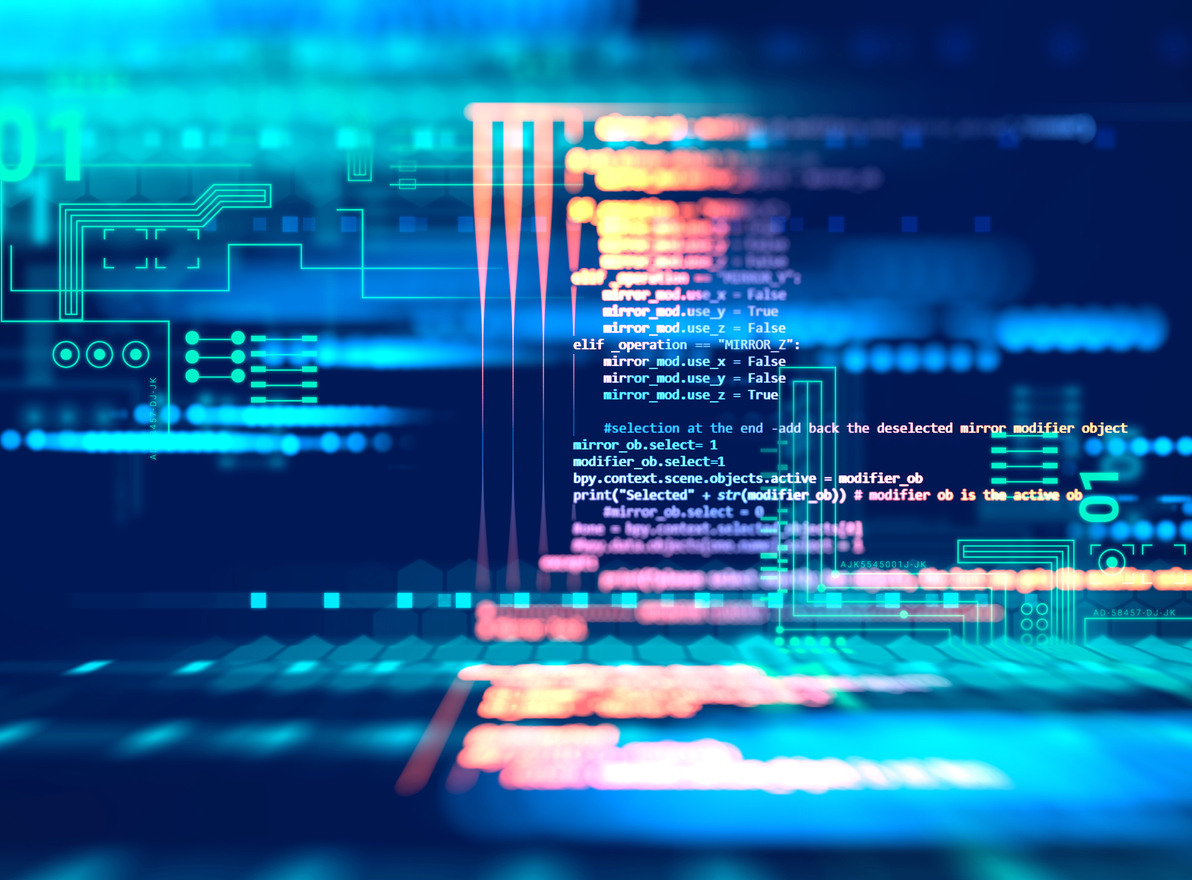
The machines have arrived. Right across healthcare, advanced analytical and cognitive technologies are sucking in data, aggregating, analyzing, then spitting out insights hidden in previously disconnected data. As they work tirelessly, we humans stand by salivating at the prospect of fast, high-quality and well-curated data to speed discovery and time to market, moving us closer to personalized, outcomes-based healthcare.
“The only way to constantly improve a system is to generate and analyze data to find solutions,” explains Dr Bertalan Mesko, the Medical Futurist. “The basic requirement of improving healthcare is everyone accessing their own medical/health data stored in semantic databases [that] generate alerts about medical issues and potential health complications.”
Mesko cites work by ElationEMR, Curemd, Drchrono, Medopad and Practice Fusion. “By measuring more data – genomics and lifestyle – we would be able to prevent conditions, delay their progress and find treatments and treatment combinations we have never used before, taking the guesswork out of drug discovery,” he says.
COGNITIVE COMPUTING IN ACTION
If we truly want to improve patient outcomes and the economics of healthcare delivery, we must move upstream and figure out which patients are at risk earlier in their disease progression and what therapeutic interventions are going to be effective, says John Piccone, Agile Insights Leader at IBM Watson Health.
IBM’s artificial intelligence system is already being used in hospitals worldwide to better inform oncologists’ decisions as part of Watson for Oncology, and grabbed headlines by diagnosing a rare leukemia at the University of Tokyo after months of fruitless effort by doctors.
Piccone outlines four areas where IBM Watson are seeing most interest and highest value:
• Identifying unmet medical need and accelerating development
• Communicating and collaborating among stakeholders
• Differentiating therapies based on outcomes, safety and economics
• Individualizing and improving care delivery.
In clinical trials, Watson can make recommendations that assist study teams in fitting studies to current patient data. For example, it can validate protocol enrollment potential against current patient populations and recommend changes in order to optimize inclusion/exclusion criteria and enhance compatibility with patient populations through statistical algorithms.
“We’ve built a very robust data supply chain,” says Piccone. “Up to 80% of time on a project can be just getting the data ready for analysis, so having the data supply chain pre-staged eliminates that work and jumps right into the analysis. You can go from 18 months to three weeks, that kind of acceleration,” says Piccone.
Cloud technology means resources can be scaled at significantly lower incremental costs, he adds. “You can substantiate a real-world evidence environment in whatever jurisdiction you need – the technology allows you to make your environment portable. Many countries won’t let you take data out of their borders – you need to bring your tech platform to that country, so you can work there.”
MAKING DATA USEFUL
With the evolution of the internet of things, future RWE data sources will be both more diverse and more comprehensive in measuring patient health. Blockchain technology, debuted with Bitcoin, will enable the secure storage of patient health data and the direct transmission of anonymized data to pharma companies.
“The number one barrier is the interoperability of EMR systems, however, as time goes on, we can develop software links that allow us to overcome that barrier,” says Ken Carson, Senior Medical Director at healthcare technology company, Flatiron Health. “Even in common-format systems, individual sites might have a different software builds so we end up having to rectify those issues.”
Standardization to a common data model to enable interoperable, transparent evidence generation ecosystem is an inevitable development, he says. “In the future, data providers, technology providers, researchers, and other RWE stakeholders will increase the value of analytics in life sciences innovation by plugging into a standardized RWE infrastructure that supports interoperability between rich and diverse data sources and analytic tools.”
Another barrier is the perception among physicians that a clinical trial is the only way to get a valid answer to a clinical question, says Carson. “Trials are expensive, time consuming and impractical for real-world clinical questions, so we need to develop the right techniques to ensure that real-world evidence is accepted by the medical community. It is not so much about a technology, rather doing the due diligence to validate our methods so that they are accepted by clinicians and RWE is seen as simply another tool in the clinical decision making process.”
ANOTHER TOOL?
Companies must remember that the technology is just a means to an end, says Robin Murray, Director RWI Technology at QuintilesIMS, and leader of the E360 technology suite. “Too many people are sold generic big data technology and then ask ‘What can I use it for?’ We need tools that allow companies’ ordinary team members to interact with complex health data. But it gets incredibly complicated; you have to understand the epidemiology, data models, data science algorithms, the maths, but it’s impossible to have that span of knowledge. Compartmentalizing is key; companies need tools that allow users to search the largest clinical data sets in near real-time using standard concepts like phenotypes and code lists, for example.”
Such tools are being developed to aid trial design and optimization (designing trials in real time), recruitment, observational study design and implementation, data visualization and predictive analytics, merging existing real-world data with trial data, health economics studies, commercial effectiveness, and much more, says Murray.
Access to data remains a limiting factor. “Capturing high-quality, real-world data is a big challenge because few health systems or countries have joined-up data, which means there’s duplication and fragmentation. While data governance is necessary, it brings massive complexity. If we could freely link data across data silos, we could develop deep insights much faster, but for very understandable reasons, we have strict data governance and privacy rules to follow.”
Murray agrees with Carson that data standardization is essential. “Work is being done on common data models and coding schemes but it is a long, arduous process. Data science algorithms are still quite immature but there has been a lot of progress and more will be made in coming years. I’d love to see a standard library of phenotypes – base-level building blocks for analysis. For example, if we want to know everyone who has diabetes, we’d have a standard definition of diabetes.”
THE HUMAN TOUCH
While the march of the machines continues, human beings will not become redundant. “Technology must combine with human elements to derive high-quality evidence,” says Carson. “The human element of analysis is a critical piece of the puzzle that AI won’t replace. I use the analogy of the airline pilot who can take control when things go wrong and make critical decisions.”
The human component is irreplaceable, says Mesko. “If we look at the history of automation, the first wave of machines in the 19th century was better at assembling things than people, while second-wave machines were better at organizing things. Today, data analytics, cognitive computers, and self-driving cars are better at pattern-recognition but humans are still superior at working with and caring for others humans. Making a diagnosis may be cheaper with cognitive computers but it is a huge question whether a robot can make an ethical decision.
“Automation will make the world better and create opportunities for those people who are clever enough to seize them, but healthcare will change,” he says. “Tasks and procedures that can be automated should and will be. Algorithms will make diagnoses based on quantifiable data in a better way than how humans reach those conclusions now. It is easy to automate the fabrication of equipment or the transportation of patients; the challenge comes when empathy and interpersonal interaction comes into play. Robots won’t approach this level of sophistication for a long time.”
IDENTIFYING THE UNIDENTIFIED
Safeguarding personal health information and ensuring privacy becomes difficult as pharma companies focus ever more on niche indications and rare diseases. However, technology-based solutions can help to de-identify and anonymize patient-level data, says Ben Hughes, VP, Strategy & Technology, Real World Evidence, at QuintilesIMS.
“Previously, the industry used simple de-identification techniques to manage privacy but we’re looking for deeper insights now, and with that comes a greater risk of reidentification. Imagine a woman in her early 50s in North Carolina who has given birth to triplets; there’s a strong likelihood she would have made the news. [But] we can use algorithm engines and machine learning to determine privacy risk and implement appropriate safeguards,” he says. Privacy and consent considerations have become increasingly critical as new machine learning and predictive analytic techniques are applied to data, says Hughes, who points to real-world data and advanced analytics being used to find undiagnosed patients. “What if we could find patients at an earlier stage using anonymous patient-level data already captured in clinical practice?
“Detailed RWD on symptomology, diagnoses, treatment history, lab tests and more – routinely collected anonymously – is a rich source that predictive analytics can exploit. Complex, subtle patterns can be seen in the data of diagnosed patients with rare conditions that can help to identify undiagnosed or misdiagnosed sufferers. This is one of the most exciting and novel technologies we’ve been using with clients in the RWE space,” he says.
This article first appeared in Trends in Real-World Evidence, created for the Data, Evidence and Access Summit (November 13-14). Pre-order the brochure here