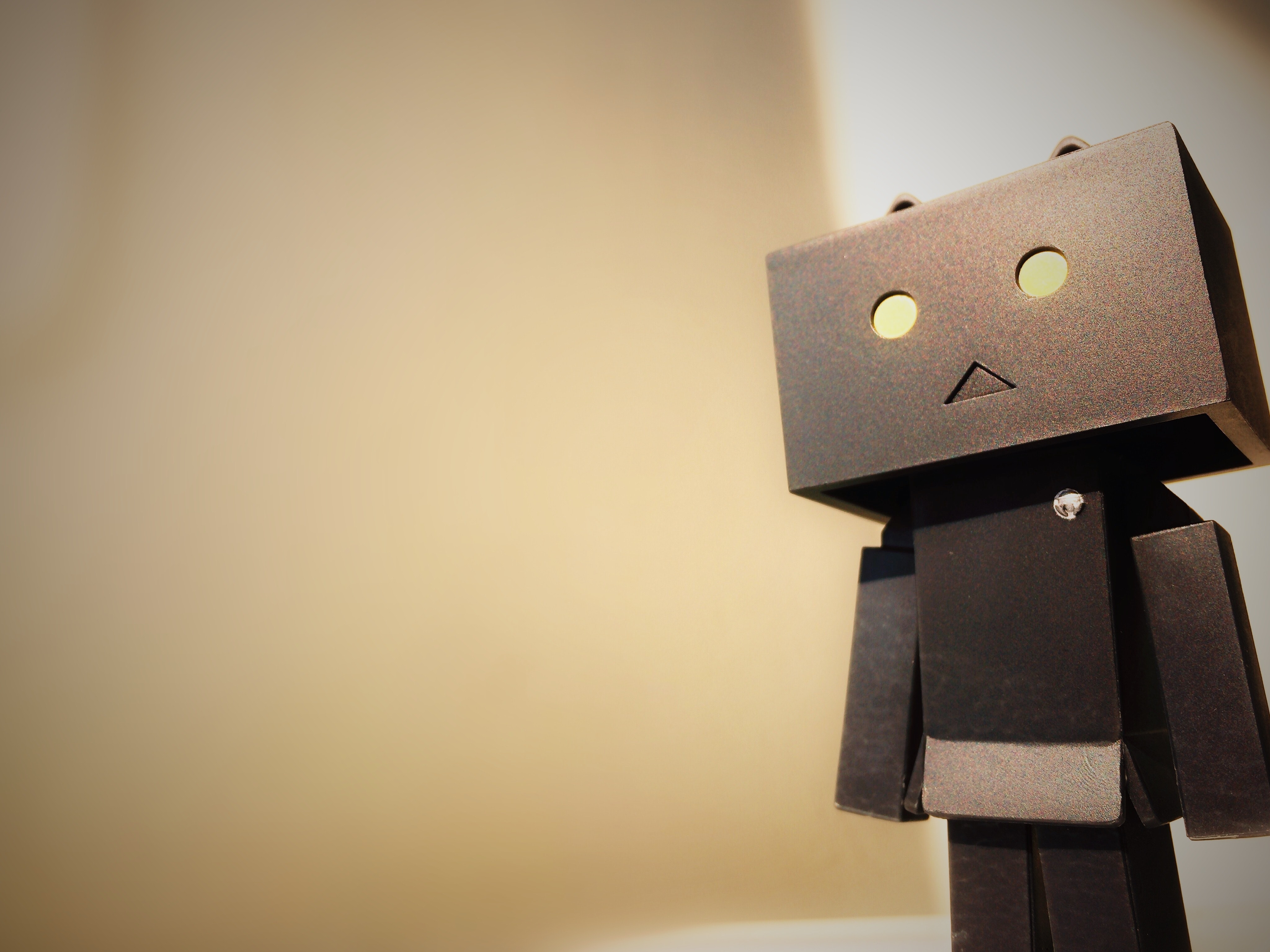

A new series on The Exponential Actuary of the Future
Forward to Part 5
Back to Part 3
The future of actuaries cannot be ascertained without looking at the macro "meta-trends" working in the larger scheme of things... In the fourth post of a new series on The Exponential Actuary of the Future, which outlines multiple scenarios for the future of the actuarial profession, we elaborate a plan of action for actuaries to remain relevant in future ...
Building the Right Outlook
Here we describe what we should do to future-proof our models, tools, and mindset, and how we should embrace exponential technologies like automated machine learning and blockchain to thrive in the fourth industrial revolution of AI.
Like Jeff Bezos, we ask ourselves: when everything changes, what doesn’t change? There will always be risk; there will always be insurable items and a need for insurance. There will always be a need for analytics and data-driven decision-making. Climate change will become a more important aspect of our daily lives and so will ageing populations. How do we structure our societies and how will economics impact how we develop our own roles?
Professions exist to give a third-party independent signal of trust to other stakeholders that the company is being honest. With blockchain and crowd wisdom taking over, trust will be disintermediated from actuaries and others to technology and the crowd. Moreover, if 3D printing eliminates scarcity and creates a post-abundance society, then won’t actuaries be a relic of the past?
Like all other human beings, we actuaries too have our cognitive biases; being ambiguity-averse, applying what we know instead of what’s best, herd mentality from jobs to corporate hierarchy. Over the past decade or so, there has been increasing interest in behavioral economics / behavioral psychology to decode what goes on in our minds when we make financial decisions. How do numbers impact us? How do we decide what to do?
In years of research, it has come out that we are consistently irrational and prone to a number of cognitive biases when taking decisions. We cannot escape from these cognitive biases (there are too many biases and they are part of how we have evolved over millennia as human beings) but we can be aware of them and “de-fang” them as it was put by Nassim Nicholas Taleb. We use many mental shortcuts (called heuristics) to take decisions and make sense of the explosion of information that we receive with our limited time and mental resources.
Utilizing opensource technologies as much as possible should be a core aspect of future plans. We are seeing a revolution brought about by opensource. No longer is it the situation that only propriety software, sitting on protected computers in some big insurer’s premises, has all the data and modeling software.
Now we have github as a coding depository where anyone can ‘fork’ existing code and modify it; there are Massive Online Open Courses (MOOCs) like Coursera, Udacity, edX, Datacamp etc. where all the latest technologies and programming are being taught for cheap prices online; We have Hadoop, R, Python, free software for database handling, web design, business intelligence and for just about anything. Instead of making, for instance, your own GLM source code, just install the GLM library in R and start applying.
The opensource trend is exponential because we don’t have to sit in our isolated corners and try to reinvent the wheel. Our macro strategy has to be leveraging technology partners and utilizing SaaS to continuously deepen our modeling expertise and utilizing white-labels as much are available to increase skill transfers. We have to aim to stand on the shoulders of giants to look beyond what others are able to see.
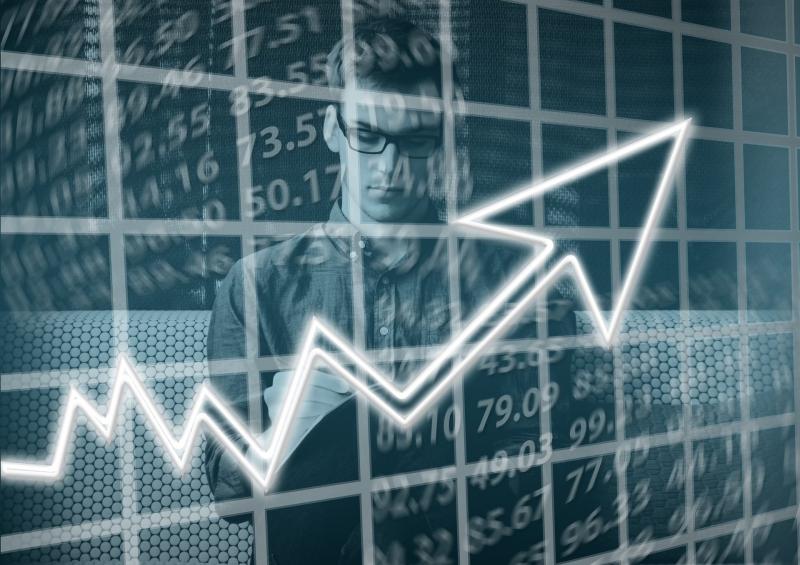
Future Proofing our Modeling Regimes
There are few fundamentals to guide modeling frameworks that will remain the same in the future. These few un-wavering fundamentals are:
- Apply both quantitative and qualitative analytics. No one model or method knows it all
- Never forget risk; almost everything can be broken down into: what core risks are you taking as an insurer or Insurtech? Model those risks
- Integrate your big-data strategy with other crucial strategies like product strategy, business plans and so on. Make holistic sense; one strategy should complement the other
- Put the customer and Artificial Intelligence (AI) at the core of everything that you do. Without better customer experience, the products won’t sell. Without putting AI at the heart of what we do, other players (especially tech disrupters) will make you redundant.
- Learn continuously; new technologies are emerging before previous ones mature. Never be afraid to try out something new and learn fast and quickly adapt. Have a futuristic vision; communicate your vision to stakeholders and invite them along for the journey.
- Combine domain expertise with AI to overcome limitations of each (domain or AI) on their own.
- Handling more types of data, not just structured in spreadsheets; more types of modeling; qualitative streaming in real time etc more schemas of data like MongoDB etc
The future-proof “Modeling Regime” in the future must be refocused from ‘doing things right’ to ‘doing the right thing’, by being more holistic and broad. The data science strategy that is suited to creating the future is broad and focuses upon the following strands and types of analytics:
- Descriptive analysis
- Predictive analytics
- Unstructured data analytics
- Big Data
- Actuarial analytics
- Enterprise Risk Management (ERM) modeling
- Qualitative profiling including emerging risks
The underlying motivation for having a broad modeling regime instead of a precise compact regime follows the thought of Peter Drucker in that ‘doing the right thing’ is more important than ‘doing things right’. Actuaries have historically over-specialized and focused on obsessive accuracy within these well-defined compartments, which means that they have no time or resources available to broaden their modeling approaches.
I suspect that this is particularly true of previous generations more so than millennial actuaries. Of course, no one actuary can do it all but together, as a profession, we can do the right thing without fragmenting into too many specializations that make us lose sight of the bigger picture.
New risks, products and liabilities are emerging and are becoming antiquated before they can even ossify. Constant revolutionizing of technology constantly keeps our social relations in uncertainty. Pre-emptive action and pro-active in-the-nick-of-time involvement is now perhaps the only way for us to go about dealing with the rapid and fast-moving present and future.
We must envision technology to automate our regular, monotonous work so that our employees are free to do work that really matters. We can keep our employees happier by freeing their time to do more strategic work as well as increase our scaling potential. Scaling potential means that we can radically increase our revenues but keep our employee count more or less on similar levels. This new automated organization is a sharp break from current insurers whose methods do not scale; there is just too much redundant bureaucratic work to do, and this creates mental drudgery and Dukkha or suffering for the employees. As these methods do not scale, doubling revenues mean almost doubling employees.
The Dukkha suffering also arises where humans are not utilized to their full potential to do creative and exciting work but are instead left to forever continue doing boring repetitive work. This model simply doesn’t work when it comes to young millennials because we want to do something meaningful instead of becoming a Sisyphus. Sisyphus is a man in Greek mythology condemned to roll a boulder up a mountain onlu for it to roll back down; and then push the boulder again to the top, endlessly and forever (Albert Camus). Despite what Albert Camus says - that “we must imagine Sisyphus happy" - we cannot imagine a Sisyphus doing repetitive monotonous work forever to be a happy employee or a happy customer.
AI is altering the actuarial landscape. AI brings to the actuarial profession a structured, consistent and unbiased way to perform actuarial work that minimizes the need for human intervention. AI, coupled with process automation and technology, will make the actuary much more productive. This is not science fiction. IBNR Robot, developed by Nicholas Actuarial Solutions, has been implemented in actual pricing and reserving work.
Based on statistical techniques including jack-knifing, runs test, hypothesis testing, Lagrange multipliers, and the method of moments, loss reserves are calculated in seconds without the need for human intervention. With the IBNR Robot, data reliability is ensured, actuarial assumptions such as development factors, tail factors and seed loss ratios are automatically selected, actuarial methodologies (paid vs. incurred data, link ratio vs. Bornhuetter-Ferguson methods) are optimally chosen, and the reserve range is automatically calibrated.
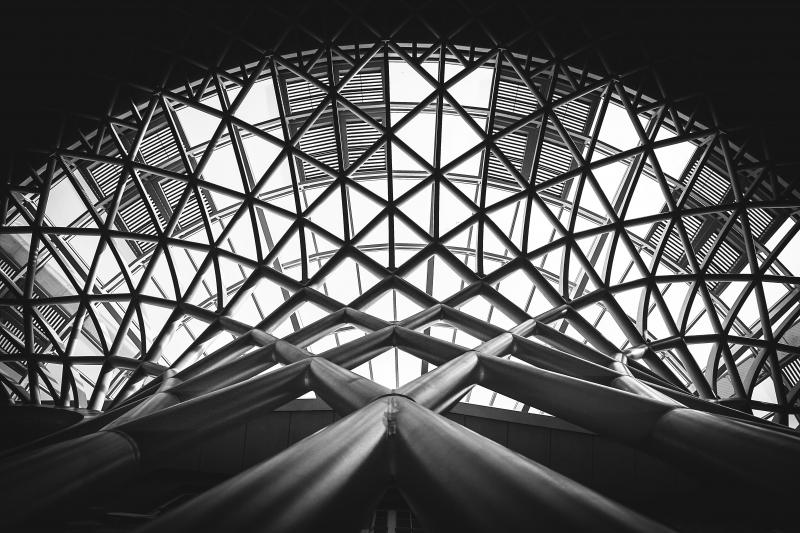
Automated Machine Learning
There is of course a broader context to automation which almost every field is subject to, and no one is free from this fear of AI apocalypse. There’s also a brighter side of automation where it will allow humans to explore ‘play’ instead of work only.
Despite the hype and glory associated with quantitative modelers like data scientists, actuaries, quants, and many others, they face a conundrum which automated machine intelligence sets out to solve. The conundrum is the gap between their training and what they should be doing compared to what they actually do. The bleak reality is most of time gets taken by data janitorial work like repetitive tasks, number crunching, sorting out data, cleansing data, understanding it, documenting the models and applying repetitive programming (spreadsheet mechanics too) and good memory to remain in touch with all of that mathematics.
What should they be doing is being creative, producing actionable insights, talking with other stakeholders to bring about concrete data-driven results, analyzing and coming up with new ‘polymath’ solutions to existing problems.
Automated machine intelligence (AML) takes care to reduce this huge gap. Instead of hiring a team of 200 data scientists, a single or few data scientists using AML can utilize fast modeling of multiple models at the same time because most of the work of machine learning is already automated by AML like exploratory data analysis, feature transformations, algorithm selection, hyper parameter tuning and model diagnostics. There are a number of platforms available like DataRobot, IBNR Robot, Nutonian, TPOT, Auto-Sklearn, Auto-Weka, Machine-JS, Big ML, Trifacta, and Pure Predictive and so on.
This way, AML frees up data scientists to be more human and less cyborg-Vulcan-human calculators. Machines are delegated to what they do best (repetitive tasks, modeling) and humans are delegated to what they do best (being creative, producing actionable insights to drive business objectives, creating new solutions and communicating them).
It is pointed out that the traditional actuarial approach of waiting until products are developed and launched and there is sufficient and credible data and only then proceeding with ratemaking is inadequate and severely limited for the challenges of today. The approach required to handle emerging landscapes of new risks, products and liabilities does not require few new algorithms but a complete overhaul of our mentalities and technical competencies.
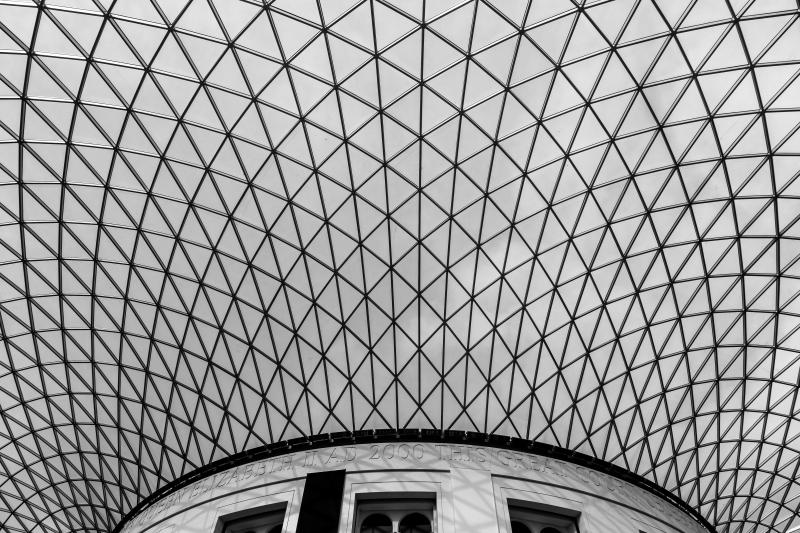
Insurtechs of the Future
To make the process even more seamless, agile, robust, invisible and as easy as a child playing, blockchain technology is used with smart contracts that execute themselves when conditions are meet. This new P2Pinsurance model is doing away with traditional premium payment using instead a digital wallet, where every member puts in their premium in an escrow-type account only to be used if a claim is made. In this model, none of the members carry an exposure greater than the amount they put into their digital wallets. If no claims are made all digital wallets keep their money. All payments in this model are done using bitcoin,further reducing transaction costs.
Teambrella claims to be the first insurer using this model based on bitcoin. Indeed, Teambrella is not alone. There are many blockchain-based startups targeting peer-to-peer insurance and other areas of human activity. Some of them are:
- Etherisc
- Insurepal
- AIgang
- Rega Life
- Bit Life and Trust
- Unity Matrix Commons
Thus, a lot of crowd wisdom is utilized in this as the insurer ‘Learns from the people, Plans with the people, Begins with what they have And Builds on what they know' (Lao Tse).
Instead of an actuary maximizing profit for the shareholders, sitting isolated from ground realities, lacking skin in the game, and having far less access to awareness (i.e., data) of people relative to their peers, this peer-to-peer approach empowers the crowd and taps in into their wisdom (instead of wisdom from books), which is far better. There are also no unfair pricing practices here like rating based on gender, pricing optimization which charges you higher if you are less likely to shift to another insurer and vice versa. The giant insurer cannot know you more than your peers, it’s as simple as that.
This same peer-to-peer insurance can be carried out on non-blockchain-based distributed ledgers too like IOTA, Dagcoins and Byteballs with the additional technological benefits of these new ledgers over current blockchains. These digital tokenization startups have the promise to radically reinvent business models where transactions, pooling and just about anything gets done for the community and by the community in an automated, fully trustworthy manner with no oppressive middlemen like governments, capitalist businesses, social institutions and so on. Peer-to-Peer Insurance is just one part of the whole program.
Smart contracts have built in conditions with them, which are automatically triggered when the contingency happens, and claims get paid instantly. The huge need for labor force with high qualifications but essentially doing clerical work is removed altogether to build a sleek, autonomous organization of the future. The oppressive middleman of ‘shareholders’ are avoided, which means that consumer interests are acted upon by providing convenience, low prices and good customer support.
In this peer-to-peer setting, the benefits go to the community instead of the shareholder. IoT provides the main source of data to these pools to develop protocols as to when to release claim payments and when not to. The same tokenization means that anyone anywhere can have access to the insurance pool instead of being limited by geography and regulations.
To summarize, in such situations of never-ending rapid changes, it is imperative for the future actuary to:
- Improve our tools. We live with increasingly complex systems and solve complex problems but many of our tools are too reductionist for handling these nuances.
- More importantly, change our mentality; qualifying as an actuary alone will not guarantee everything. Many tools will likely be outdated once an aspiring actuary ultimately becomes a fellow. Familiarity bias, where we continue using tools which we know rather than what is the best, coupled with being ambiguity-aversion may prove to be our Achilles’ heel. We need to learn more diverse subject areas and viewpoints, strengthen qualitative understanding, and be more pro-active and better at communication. Continuous learning has to be in our bones.
- Actuarial modelling projects naturally fall into the category of supervised learning, with tasks such as insurance contract pricing or pension scheme valuation naturally fitting into this framework. This makes supervised learning tasks a natural place for actuaries to initially explore machine learning techniques.
- Start with what you know; innovate within our comfort zone in supervised learning in current insurer jobs and then go ahead into unsupervised and other careers etc.
- No one can do it all. Share, build team work, collaborate across multi-disciplinary teams